CASE STUDY: Enhancing Factuality in LLMs
Discover how Welo Data partnered with a reputed technology brand to help generate factually accurate responses.
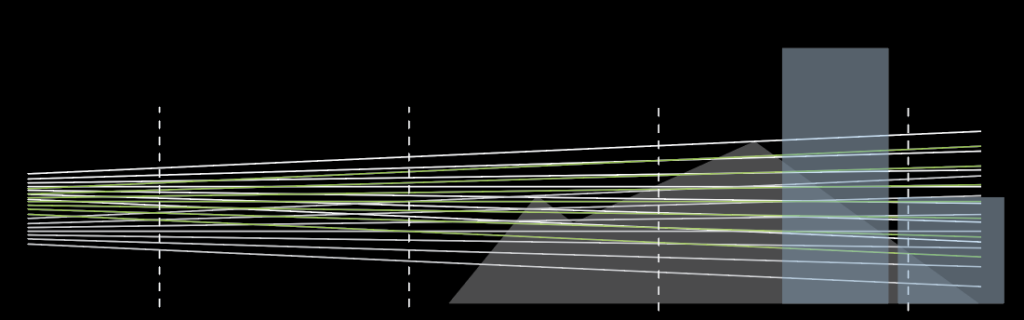
This case study focuses on how Welo Data partnered with a large technology firm to improve the factuality and accuracy of responses of large language models (LLMs). We implemented rigorous data annotation and quality control processes to improve LLMs’ ability to generate content consistent with factual information and world knowledge.
Scroll 👇 to read the case study. Learn how we partnered with a reputed technology brand to help generate factually accurate responses.
The Client
The client is a global technology company, offering a wide range of AI-powered products, including large language models (LLMs) that deliver accurate and reliable information. These models make search-related tasks easier and more intuitive for users across various applications.
The client aims to improve the user experience by providing fact-based, credible responses to minimize the risk of misinformation. They sought Welo Data’s expertise to enhance the factual accuracy of their LLMs. The primary issue was the presence of hallucinations that were negatively impacting user trust.
The Challenge
The client manages extensive datasets, which require meticulous verification to ensure their accuracy and reliability. However, they faced challenges with their LLMs generating inaccurate or misinformation content, which was a major concern for user trust in important areas like health and financial advice.
While the models were advanced, they did not have any mechanism to ensure that the responses were credible and came from verified sources. In our case, our assignment was to devise a solution that included human raters capable of analyzing the accuracy and factuality of LLM outputs, detecting hallucinations, and enhancing the level of responses.
The Solution
The technology firm asked Welo Data for help creating a solution centered on human credibility assessment and verification. The goal was to ensure that the LLM outputs aligned with credible and verified sources of information.
Welo Data focused on improving the quality of AI training data by using skilled human raters. We implemented a comprehensive strategy centered on factuality testing to tackle these issues. This involved:
- Data Annotation and Labeling: With around 1000 raters from our global workforce of over 500,000 experts, we annotated datasets to ensure they were relevant and accurate. This human-in-the-loop approach enhanced the quality of the training data.
- Human Rater Evaluation: Nearly 1,000 raters were trained to verify the accuracy of the LLM outputs using strict guidelines. We used the Welocalize Competency Matrix to identify the skills required for successful factuality tasks. These raters were tasked with cross-checking information against trusted sources to ensure that no misinformation or hallucination occurred.
- Training and Qualification: Raters were trained using a detailed factuality task template. Sensitive topics were given special attention to ensure accurate and reliable responses.
- Factuality Testing: The system also included regular factuality testing of LLM responses and flagging content that contained hallucinations or inaccuracies for further review. This includes cross-referencing generated content with reliable sources to verify facts.
- Quality Control Measures: Advanced monitoring systems were put in place to track the performance of LLMs continuously. Raters were scored weekly based on sample evaluations of their work and received feedback on their accuracy. This ensured that any inaccuracies could be identified and corrected promptly.
The Results
The Welo Data approach to training human raters and maintaining quality control has improved the LLM’s ability to generate factually accurate responses with further work planned. The initial data suggests:
- Improved Accuracy: Raters’ assessments have reduced hallucinations noticeably, and the LLM’s accuracy in providing fact-checked information has improved significantly.
- Positive Correlation with Training: Raters who performed well in our assessments consistently delivered high-quality factual evaluations that aligned with the client’s objectives.
- Client Satisfaction: While the project is still in its early stages, the client has expressed satisfaction with the initial results, noting the effectiveness of our methods in reducing misinformation.
Enhancing Factuality in Large Language Models (LLMs)
Key Challenges
- Inaccurate information generated by LLMs
- User distrust due to lack of factual accuracy
Welo Data Solutions
- Data Annotation and Labeling Human
- Raters Skill-Based Assessment
- Factuality Testing
- Rigorous Quality Control
- Training and Refinement
Conclusion
This case study highlights the importance of factual accuracy in AI applications. The collaboration between Welo Data and the client illustrates a successful strategy for enhancing factuality in LLMs. Welo Data demonstrated its commitment to delivering high-quality AI solutions by implementing structured frameworks, using skilled human raters, and maintaining rigorous quality control measures.