CASE STUDY: Improving Helpfulness in LLMs
Discover how Welo Data improved the relevance and alignment of LLM responses with user intent.
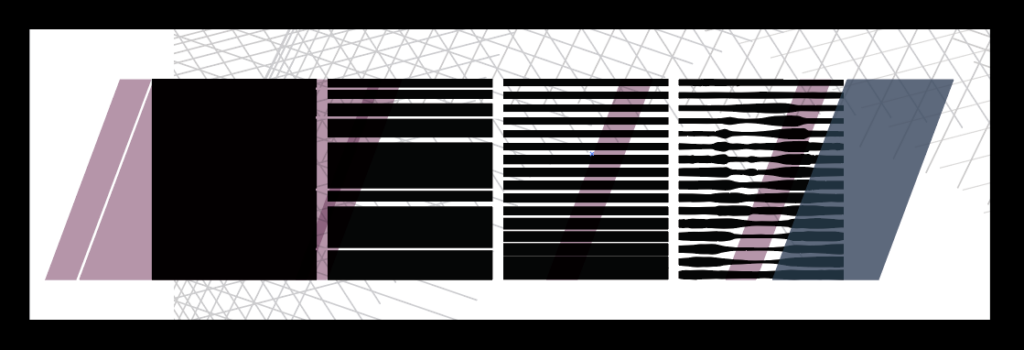
This case study focuses on Welo Data’s collaboration with a leading technology firm to enhance the helpfulness of their large language models (LLMs).
The client needed to improve the ability of their AI systems to assist users effectively. By enhancing the helpfulness of their LLMs, the client aimed to create a more engaging user experience and increase overall satisfaction with their AI-driven solutions.
Scroll 👇 to read the case study. Learn how we partnered with a reputed brand to improve helpfulness in large language models.
The Client
The client is a renowned AI and technology company known for pushing the boundaries of AI and machine learning. With a strong emphasis on innovation, the company offers a wide range of AI-driven tools integral to users worldwide, from search engines to personal assistants. They aim to enhance the user experience by ensuring their Large Language Models (LLMs) deliver accurate information and align closely with user intent.
Recognizing the growing importance of AI helpfulness, they wanted to optimize how their LLMs interact with users to ensure relevant and genuinely helpful responses. The client turned to Welo Data for their expertise in data quality, human evaluation, and prompt engineering.
The Challenge
While the LLMs were generally accurate, they struggled to consistently deliver helpful or relevant responses to user queries. They often provided overly complex or irrelevant information, frustrating users and creating inefficiencies in search-related tasks.
The client needed a system to ensure the LLMs understood user intent more effectively and responded with concise, actionable information. The challenge was to streamline the LLM’s output to make them more helpful and improve responses’ relevance without compromising accuracy.
The Solution
Welo Data stepped in to address this challenge. We offered a solution focused on human evaluation, customized training, and continuous performance monitoring to improve the helpfulness of LLM outputs.
Key steps taken include:
- Human Rater Evaluation: We deployed a team of trained human raters to evaluate the helpfulness of the LLM responses. Raters assessed how well the output aligned with the user’s intent and whether the response was concise and relevant to the query.
- Targeted Training and Assessments: Using the Welo Data Competency Matrix, raters were trained on specific task guidelines to assess helpfulness effectively. Special assessments were also developed to ensure the raters can accurately evaluate the usefulness of responses based on user queries.
- Continuous Monitoring and Feedback: The raters’ performance was regularly monitored to avoid any consistency in their evaluations. We used a system of feedback loops to refine the process and ensure that unhelpful responses were flagged and corrected over time.
- User Intent Alignment: The solution’s core was to ensure that the LLM understood and responded to user intent. The LLM’s ability to provide helpful responses improved significantly through prompt engineering and refinement, focusing on delivering concise and actionable information to users.
Their Results
The project is still in its early stages, having been live for just three months, but initial results are encouraging:
- Improved Helpfulness Scores: Early data indicates a positive correlation between rater assessment performance and high scores in helpfulness tasks. This suggests that Welo Data’s training is effectively enhancing LLM performance.
- User Feedback: Users reported increased satisfaction with the AI’s ability to provide relevant and concise information, indicating a shift towards more helpful interactions.
- Faster Deployment: With improved data quality and speedier testing cycles, the firm brought new features to market more quickly.
Improving Helpfulness in Large Language Models (LLMs)
Key Challenges
- LLMs provided unclear or unhelpful responses
- User dissatisfaction due to lack of relevant information
Welo Data Solutions
- Human Raters Skill-Based Evaluation
- Targeted Training and Assessments
- Continuous Monitoring and Feedback
- User Intent Alignment
Conclusion
This case study highlights how Welo Data’s expertise in data quality and human evaluation can boost the helpfulness of large language models. Through human evaluation, targeted training, and continuous monitoring, Welo Data improved the relevance and alignment of LLM responses with user intent. Early results show increased user satisfaction and faster feature deployment, highlighting the approach’s effectiveness.