Strengthening AI Content Integrity Through Human Evaluation
A global tech company partnered with expert evaluators to train a journalistic LLM focused on accuracy, credibility, and readiness for real-world media use.
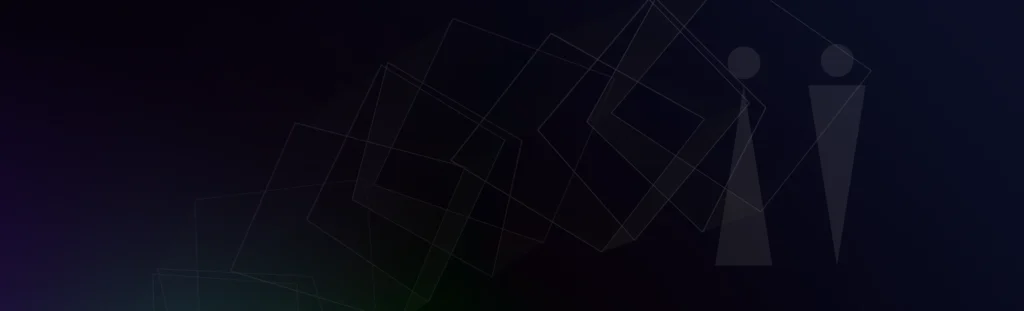
Summary
A major technology company set out to develop a large language model (LLM) capable of generating reliable, professional-grade journalistic content. The stakes were high: this model would support core business goals around AI adoption in media and information spaces.
Our team was brought in to support evaluation and help ensure content accuracy, editorial quality, and long-term scalability.
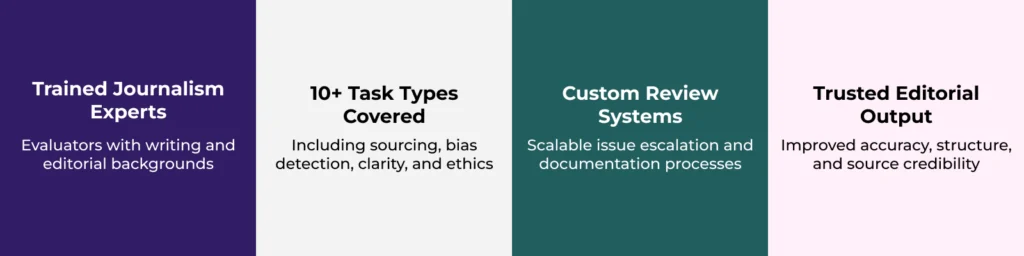
The Client
The client is a global technology company investing in LLMs tailored to specific real-world use cases. In this instance, they sought to create a model that could assist in news article creation while maintaining the integrity, factuality, and clarity that readers expect from trusted media outlets.
Challenge
At its core, the project aimed to teach an LLM how to “think like a journalist.” This included understanding:
- What makes a source trustworthy
- What context belongs in a story, and what doesn’t
- How to write clearly, factually, and without bias
- Where to draw the line between reporting and editorializing
The main challenge wasn’t just technical. Many task types were across multiple projects, each targeting a slightly different piece of the journalism puzzle: headlines, ethics, structure, sourcing, or sensitivity.
Solution
Our team of research content writers, experienced in journalism, writing, and editorial review, was embedded across all major task types. These included:
- Author Topics: Reviewing whether short phrases accurately describe a person’s relevance to a story
- Side-by-Side Reviews: Comparing AI articles to source press releases to grade structure, factual accuracy, and grammar
- Diff Con and Topic Specialist: Judging whether quotes came from direct sources and whether sources were credible subject-matter experts
- Pinpoint and Magi: Rating how well the model responded to prompts and provided useful background information
- News Labels and Idea Tasks: Checking bias, opinion, and effectiveness of new content suggestions
We also created an internal escalation and documentation system to flag unclear issues, clarify expectations, and share best practices. These protocols became a de facto quality control process, which our client appreciated.
Results / Business Outcomes
Our work directly supported the development of a more accurate, reliable, and scalable editorial AI product.
Key outcomes include:
- The model’s improved clarity, accuracy, and sourcing brought it closer to deployment in real-world editorial workflows, supporting the client’s goal of building AI for high-trust use cases.
- Expansion into new task types and evaluation areas underscored the project’s strategic value within the client’s broader AI roadmap.
- The internal review systems and escalation protocols we developed enabled scalable, consistent quality control and faster iteration cycles.
- Embedding journalistic standards into the training process helped reduce the risk of factual errors, bias, and credibility issues.
- The model is now better positioned for use in newsroom partnerships, content platforms, or other media-facing applications.
Why It Matters
This project directly supported the client’s strategic goals around building responsible, high-impact AI products for the media space.
Creating a journalistically sound LLM offers clear business value in an era of misinformation and low-quality AI-generated content.
A model trained with human oversight and editorial standards provides:
- A competitive advantage by positioning the client as a leader in ethical, trustworthy AI
- A foundation for building trust with media organizations and enterprise partners through adherence to professional standards
- Protection against reputational and legal risks by reducing the likelihood of biased or inaccurate content
- Scalable systems for quality control that maintain consistency and speed without relying on rigid frameworks
Training a Trusted Journalistic LLM for Real-World Use
Key Challenges
- Inconsistent quality and factual accuracy in AI-generated news content
- Lack of editorial context and source credibility in outputs
- No clear process for scaling human-in-the-loop evaluation
Welo Data Solutions
- Embedded trained evaluators with journalism and writing expertise
- Created scalable quality control and issue escalation systems
- Designed and executed task-specific evaluations across sourcing, clarity, and ethics
- Helped improve model accuracy and structure across evolving task types
Conclusion
This project played a key role in the client’s strategy to build trustworthy, high-impact AI for complex content environments. What began as content evaluation became a deeper partnership. By embedding trained evaluators into the development process, we helped ensure the model reflected core journalistic values: accuracy, authority, and accountability.
We filled process gaps, created scalable quality systems, and adapted to shifting priorities, bringing clarity, structure, and subject-matter expertise where it was needed most.
The result is a stronger, more credible model that supports the client’s long-term goals in media and AI, and positions them to lead in high-trust applications.